Agenda
Signal Processing Seminar
- Friday, 7 December 2018
- 10:00-10:45
- LB 1.220 van der Poelzaal
Image Reconstruction Using Training Images
Per Christian HansenTechnical University of Denmark
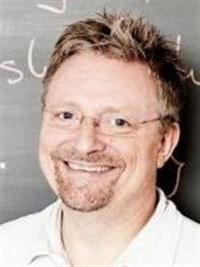
Priors are essential for computing stable solutions to ill-posed problems, and they take many different forms. Here we consider priors in the form of cross-section images of the object, and this information must be used in a fast, reliable, and computationally efficient manner. We describe an algorithmic framework for this: From a set of training images we use techniques from machine learning to form a dictionary that captures the desired features, and we then compute a reconstruction with a sparse representation in this dictionary. We describe how to stably compute the dictionary through a regularized non-negative matrix factorization, and we study how this dictionary affects the reconstruction quality. Simulations show that for textural images our approach is superior to other methods used for limited-data problems.