Agenda
PhD Thesis Defence
- Friday, 7 December 2018
- 12:30-13:30
- Aula Senaatszaal
Image formation for future radio telescopes
Shahrzad Naghibzadeh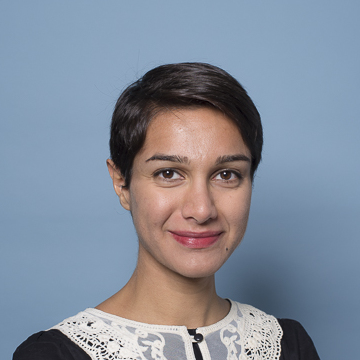
Fundamental scientific questions such as how the first stars were formed or how the universe came into existence and evolved to its present state drive us to observe weak radio signals impinging on the earth from the early days of the universe. During the last century, radio astronomy has been vastly advancing. Important discoveries on the formation of various celestial objects such as pulsars, neutron stars, black holes, radio galaxies and quasars are the result of radio astronomical observations. To study celestial objects and the astrophysical processes that are responsible for their radio emissions, images must be formed. This is done with the help of large radio telescope arrays.
Next generation radio telescopes such as the Low Frequency Array Radio Telescope (LOFAR) and the Square Kilometer Array (SKA), bring about increasingly more observational evidence for the study of the radio sky by generating very high resolution and high fidelity images. In this dissertation, we study radio astronomical imaging as the problem of estimating the sky spatial intensity distribution over the field of view of the radio telescope array from the incomplete and noisy array data. The increased sensitivity, resolution and sky coverage of the new instruments pose additional challenges to the current radio astronomical imaging pipeline. Namely, the large amount of data captured by the radio telescopes cannot be stored and needs to be processed quasi-realtime.
Many pixel-based imaging algorithms, such as the widely-used CLEAN [3] algorithm, are not scalable to the size of the required images and perform very slow in high resolution scenarios. Therefore, there is an urgent need for new efficient imaging algorithms. Moreover, regardless of the amount of collected data, there is an inherent loss of information in themeasurement process due to physical limitations. Therefore, to recover physically meaningful images additional information in the form of constraints and regularizing assumptions are necessary. The central objective of the current dissertation is to introduce advanced algebraic techniques together with custom-made regularization schemes to speed up the image formation pipeline of the next generation radio telescopes.
Signal processing provides powerful tools to address these issues. In the current work, following a signal processing model of the radio astronomical observation process, we first analyze the imaging system based on tools from numerical linear algebra, sampling, interpolation and filtering theory to investigate the inherent loss of information in the measurement process. Based on these results, we show that the imaging problem in radio astronomy is highly ill-posed and regularization is necessary to find a stable and physically meaningful image. We continue by deriving an adequate model for the imagingproblem in radio interferometry in the context of statistical estimation theory. Moreover, we introduce a framework to incorporate regularization assumptions into the measurement model by borrowing the concept of preconditioning from numerical linear algebra.
Radio emissions observed by radio telescopes appear either as distributed radiation from diffuse media or as compact emission from isolated point-like sources. Based on this observation, different source models need to be applied in the imaging problem formulation to obtain the best reconstruction performance. Due to the ill-posedness of the imaging problem in radio astronomy, to guarantee a reliable image reconstruction, propermodeling of the source emissions and regularizing assumptions are of utmost importance. We integrate these assumptions implementing a multi-basis dictionary based on the proposed preconditioning formalism.
In traditional radio astronomical imaging methods, the constraints and priormodels, such as positivity and sparsity, are employed for the complete image. However, large radio sky images usually manifest individual source occupancy regions in a large empty background. Based on this observation, we propose to split the field of view into multiple regions of source occupancy. Leveraging a stochastic primal dual algorithm we apply adequate regularization on each facet. We demonstrate the merits of applying facet-based regularization in terms of memory savings and computation time by realistic simulations.
The formulation of the radio astronomical imaging problem has a direct consequence on the radio sky estimation performance. We define the astronomical imaging problem in a Bayesian-inspired regularized maximum likelihood formulation. Based on this formalism, we develop a general algorithmic framework that can handle diffuse as well as compact source models. Leveraging the linearity of radio astronomical imaging problem, we propose to directly embody the regularization operator into the system by right preconditioning. We employ an iterative method based on projections onto Krylov subspaces to solve the subsequent system. The proposed algorithmis named PRIor-conditioned Fast Iterative Radio Astronomy (PRIFIRA). We motivate the use of a beamformed image as an efficient regularizing prior-conditioner for diffuse emission recovery. Different sparsity-based regularization priors are incorporated in the algorithmic framework by generalizing the core algorithm with iterative re-weighting schemes.
We evaluate the performance of PRIFIRA based on simulated measurements as well as astronomical data and compare to the state-of-the-art imaging methods. We conclude that the proposed method maintains competitive reconstruction quality with the current techniques while remaining flexible in terms of different regularization schemes. Moreover, we show that the imaging efficiency can be greatly improved by exploiting Krylov subspace methods together with an appropriate noise-based stopping criteria.
Based on the results from this thesis we can conclude that with the help of advanced techniques from signal processing and numerical linear algebra, customized algorithms can be designed to tackle some of the challenges in the next generation radio telescope imaging. We note that since radio interferometric imaging can be considered as an instance of the broad area of inverse imaging problems, the numerical techniques as well as regularization methods developed in this dissertation have a direct impact on many different imaging application areas, such as biomedical and geophysics/seismic imaging.
Additional information ...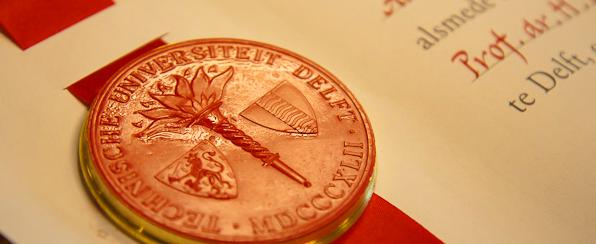
Agenda
- Thu, 4 Sep 2025
- 10:00
- Aula Senaatszaal
PhD Thesis Defence
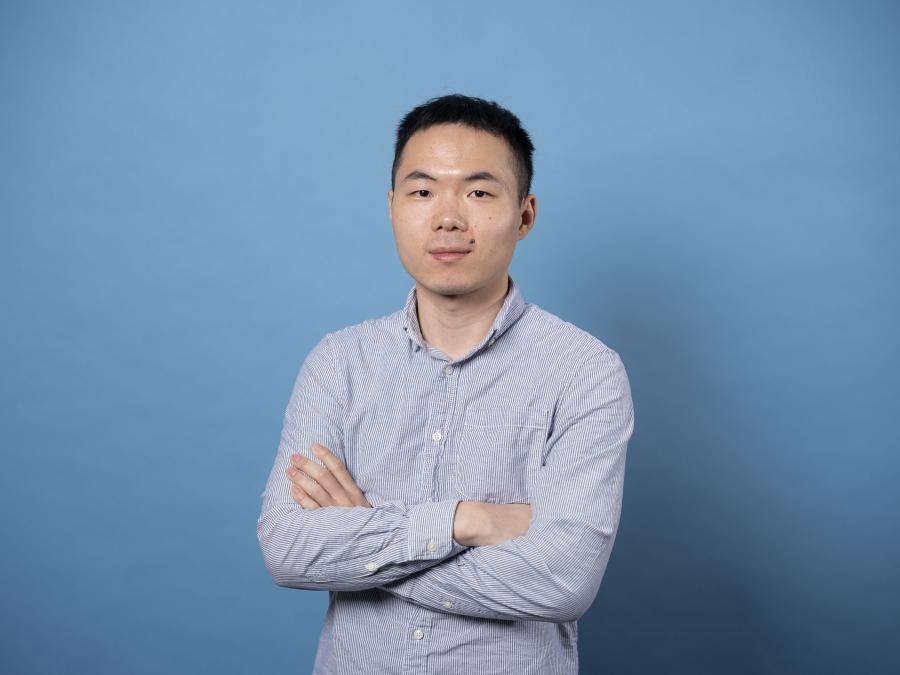
Leiming Du
Sintering Fundamentals of Nano-Metallic Particle Interconnects
- Wed, 10 Sep 2025
- 17:30
- Aula Senaatszaal
PhD Thesis Defence
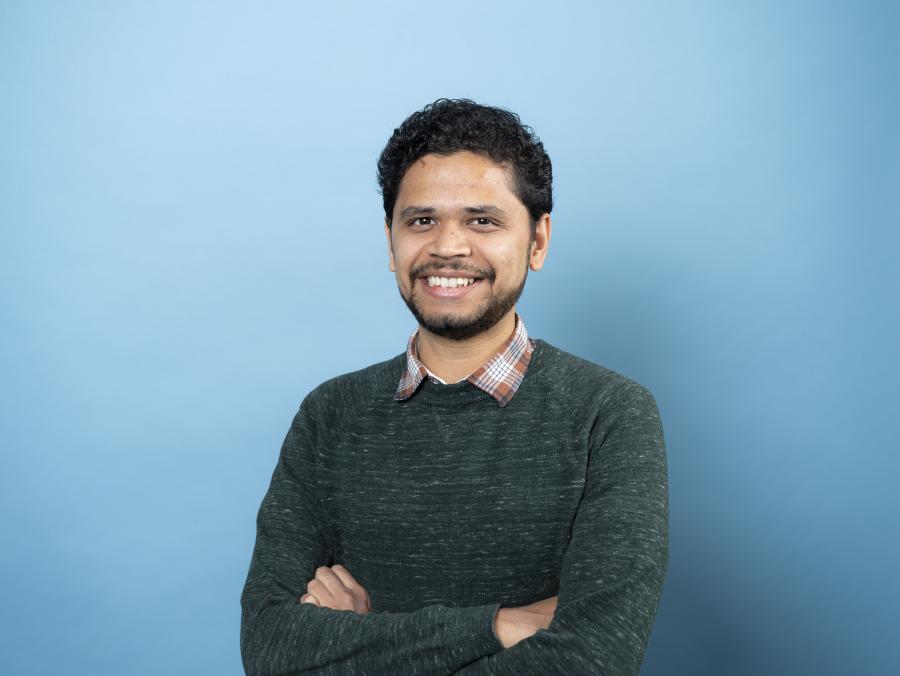
Adwait Inamdar
Digital twin-based health monitoring of microelectronics
- Thu, 18 Sep 2025
- 09:03
- Aula Senaatszaal
PhD Thesis Defence
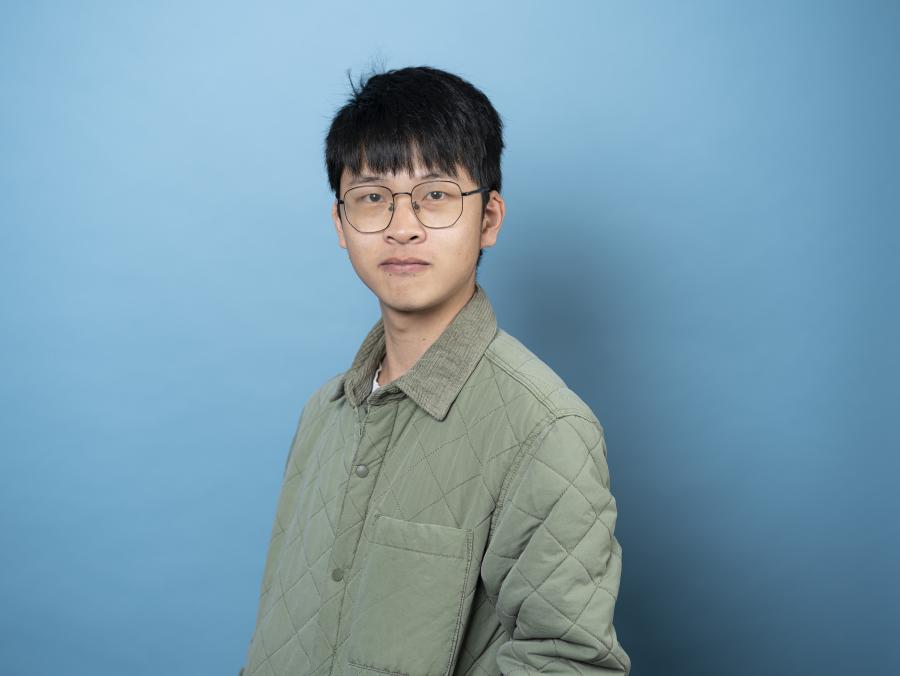
Changheng Li
Multi-Microphone Signal Parameter Estimation in Various Acoustic Scenarios
Low Complexity Approaches Utilizing Temporal Information