Single Cell and Cell-Type Resolution Bi-Directional Neural Interface for an Artificial Retina (BiNIfAR)
Current brain-machine interfaces (BMIs) provide coarse communication with the target neural circuitry because they fail to respect its cellular and cell-type specificity. Instead, they indiscriminately activate or record many cells at the same time and provide only partial restoration of lost abilities. Next-generation neural interfaces for clinical or investigational devices will need application-specific integrated circuits (ASICs) enabling massively parallel bidirectional microelectrode arrays (MEAs). In this project, we focus on the development of an ASIC capable of recording and stimulating neurons at the resolution of single-cell and single spikes. To do so, we will develop (i) hardware-friendly compression algorithms to deal with the data deluge problem in massively parallel interfaces, (ii) circuits for digital active pixels for maximum area efficiency, (iii) strategies for artifact-free stimulation, and (iv) event-driven communication protocols for low power data movement. The ASIC will be validated within the context of an artificial retina being developed at Stanford University. An artificial retina is a device that replaces the function of retinal circuitry lost to disease. In principle, a device able to reproduce the natural pattern of activation of the ganglion cells in the retina, using electrical stimulation, and transmitting this neural signal to the brain, would restore vision in the patient. Because the retina is relatively well understood and easily accessible, it is an ideal neural circuit in which to develop a BMI that can approach or exceed the performance of the biological circuitry by interfacing with neurons at their native resolution. Moreover, it serves an important clinical need (i.e. to cure blindness), for which today’s technology fails at providing a definitive solution.
The main obstacle is that there are many types of cells in the retina that deliver distinct visual signals to different targets in the brain (see Fig. 1a). Hence, the distinct cell types must be addressed independently to re-create the neural code. For example, simultaneous activation of so-called ON and OFF type cells at a given location would send conflicting “messages” to the brain, indicating both a light increase and a light decrease, at the same retinal location at the same time. Present-day retinal prostheses make no attempt to distinguish distinct cells or cell types, and therefore produce a non-specific, and thus profoundly scrambled, retinal signal of exactly this kind. Because the different cell types are intermixed in the neural circuitry of the retina, this requires cellular resolution interfaces with high channel counts to recreate complex patterns in the neural network like the one being developed in this project (see Fig. 1b). "
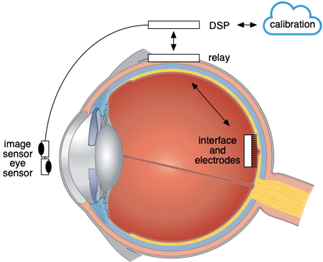
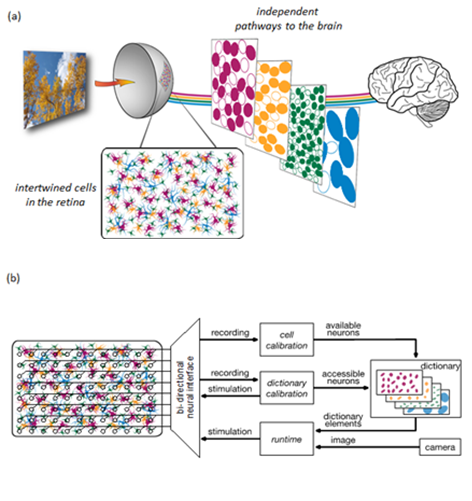
Project data
Researchers: | Dante Muratore, Yi-Han Ou-Yang, Bakr Abdelgaliel |
---|---|
Starting date: | November 2020 |
Closing date: | October 2024 |
Funding: | 239 kE; related to group 239 kE |
Sponsor: | Sector Plan |
Partners: | E.J. Chichilnisky, Stanford University |
Contact: | Dante Muratore |