MSc thesis project proposal
Epilepsy diagnosis using multimodal machine learning
Project outside the university
Erasmus MCEpilepsy is a common neurological disorder characterized by the occurrence of seizures. These attacks can be provoked by, for example, lack of sleep, alcohol consumption or fever, but can also occur without a clear cause. Making the diagnosis is sometimes difficult, especially if no good description of the attack is available. In addition, an epileptic attack can also be a one-off, without a recurrence ever occurring and the diagnosis of epilepsy being made. This uncertainty can lead to anxiety among patients and has consequences for many aspects of their daily lives, such as the performance of their profession and their fitness to drive. It is also insufficiently clear which patients after a first seizure would immediately benefit from starting anti-epileptic drugs, and in which patients a wait-and-see policy can be safely pursued.
An EEG can help diagnose epilepsy. Ideally, a seizure is captured on EEG, making the diagnosis certain. However, this is logically rare if the attack frequency is low. Instead, attention is now focused on the presence of interictal epileptiform abnormalities, specific wave patterns that occur in the EEG between seizures and are associated with an increased risk of recurrence. However, these abnormalities do not occur in every EEG of a patient with epilepsy. Previous research has already shown that it is possible to use machine learning techniques to achieve good classification, even in EEGs without epileptiform abnormalities.
In this project we aim to build on this previous research and determine whether it is possible to accurately distinguish between patients with epilepsy and controls with a different diagnosis using multimodal machine learning. We use both the EEG and clinical information. On the one hand, the aim is to improve the information and treatment of patients with epilepsy, because we can more quickly assess who will benefit from starting anti-epileptic drugs. On the other hand, by visualizing the features on which a machine learning algorithm bases its classification, we hope to gain more insight into the underlying neurological mechanisms that contribute to the risk of epilepsy.
Contact
dr.ir. Justin Dauwels
Signal Processing Systems Group
Department of Microelectronics
Last modified: 2023-12-10
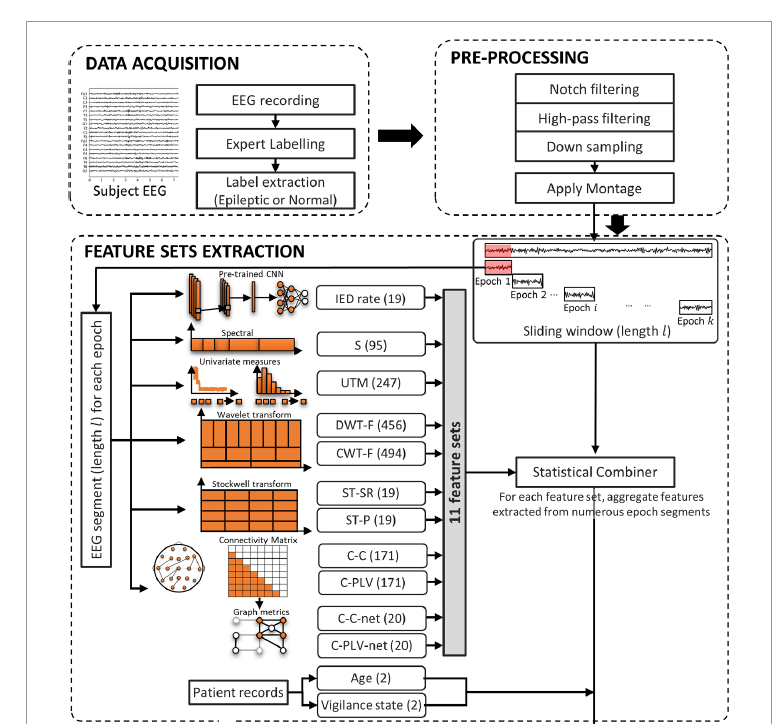