EE4675 Object classification with radar
While the idea of gaining information about the surrounding environment using electromagnetic waves is a relatively mature concept (a radar essentially!), a tremendous amount of research activities have recently coupled the analysis of data from EM sensors with techniques that can teach them to recognise automatically objects of interest.
What natural or manmade objects are moving around, and what are they doing? Can we infer something about their behaviour or intention from such movements? These are an example of the “big questions” which data and clever signal processing from EM sensors can address. Applications range from the analysis of human gait and movements to the discrimination of birds vs Unmanned Aerial Vehicles (drones), to the identification of the subtle gestures people produce to interact with smart objects, amongst others.
All these applications have in common two main areas of expertise: one has to do with understanding and processing the information encoded into the sensors’ signals (radar signal processing and data format); one has to do with the techniques to interpret this information automatically to make decisions (we could call this applied machine learning).
In this course, we aim to provide a comprehensive introduction and explanation of both areas from an engineering application perspective, with the objective to learn how to use these tools for real-life applications in radar engineering.
The course is broadly divided into two parts:
- Part 1 will build on fundamentals of radar & microwave sensing courses to investigate the concept of micro-Doppler signatures, focusing on its modelling, the signal processing for its characterisation, and its relationships with the physical movement of objects and therefore their identity. Can we understand from the EM sensors data how the different parts of human bodies or manmade objects move?
- Part 2 will introduce machine learning techniques for extraction of information from sensors’ data (feature extraction), for their selection, and for classification of objects of interest. Conventional supervised learning techniques will be presented (for example Nearest Neighbours and Support Vector Machine classifiers), along with some of the more recent data-driven approaches based on neural networks. The diversity of formats of the radar data for classification will also be considered, including micro-Doppler signatures, High Resolution Range Profiles, polarimetric information, and kinematic model-based information.
Teachers
dr. Francesco Fioranelli
Bistatic/multistatic radar systems; micro-Doppler signature characterization and classification.
Last modified: 2023-11-02
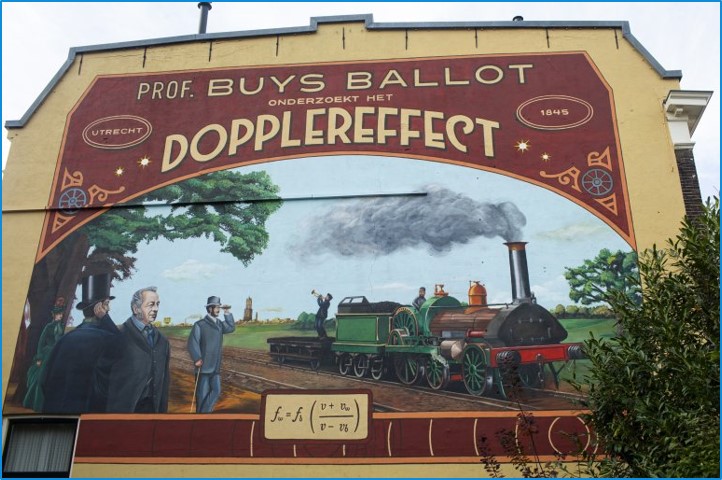
Details
Credits: | 4 EC |
---|---|
Period: | 0/0/0/4 |
Contact: | Francesco Fioranelli |